Testing AI or Not: How Well Does an AI Image Detector Do Its Job?
You may recall earlier this year when many social media users were convinced pictures of a “swagged out” Pope Francis—fitted with a white puffer jacket and low-hanging chain worthy of a Hype Williams music video—were real (they were not).
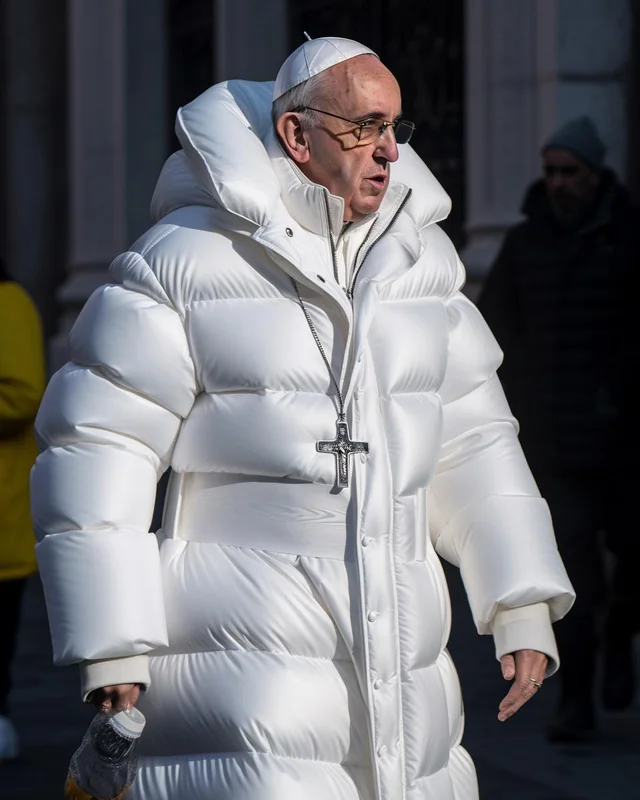
These images were the product of Generative AI, a term that refers to any tool based on a deep-learning software model that can generate text or visual content based on the data it is trained on. Of particular concern for open source researchers are AI-generated images.
DALL-E, Stable Diffusion, and Midjourney—the latter was used to create the fake Francis photos—are just some of the tools that have emerged in recent years, capable of generating images realistic enough to fool human eyes. AI-fuelled disinformation will have direct implications for open source research—a single undiscovered fake image, for example, could compromise an entire investigation.
Earlier this year, the New York Times tested five tools designed to detect these AI-generated images. The tools analyse the data contained within images—sometimes millions of pixels—and search for clues and patterns that can determine their authenticity. The exercise showed positive progress, but also found shortcomings—two tools, for example, thought a fake photo of Elon Musk kissing an android robot was real.
Bellingcat sought to repeat this experiment with open source research in mind. We tested one of these tools- AI of Not – on 200 images: half of them real, half AI-generated. In particular we wanted to understand how effective the tool was at detecting images with watermarks and images which have been compressed – two specific challenges often faced by open source researchers:. The results show a technology suited to navigating watermarks but that struggles with images that have been compressed.
The Tool
The tool is called AI or Not. Developed by Optic, an American tech company founded by former Google product director Andrey Doronichev, it “uses advanced algorithms and machine learning techniques to analyse images and detect signs of AI generation.” It has been trained on DALL-E, Midjourney, Stable Diffusion, generative adversarial networks and face image generators.
The company states that the tool is designed to provide highly accurate results.
The Method
For the test, Bellingcat fed 100 real images and 100 Midjourney-generated images into AI or Not. The real images consisted of different types of photographs, realistic and abstract paintings, stills from movies and animated films and screenshots from video games. The Midjourney-generated images consisted of photorealistic images, paintings and drawings. Midjourney was programmed to recreate some of the paintings used in the real images dataset.
The AI image dataset is available here. The real image dataset is available here. What follows are the results.
First Impression
AI or Not was highly accurate during the first round of identifications.
It was particularly adept at identifying AI-generated images — both photorealistic images and paintings and drawings.
AI or Not successfully identified visually challenging images as having been created by AI.
For example, someone without professional art training might struggle with correctly identifying this modernist-style mural as having been generated by Midjourney:
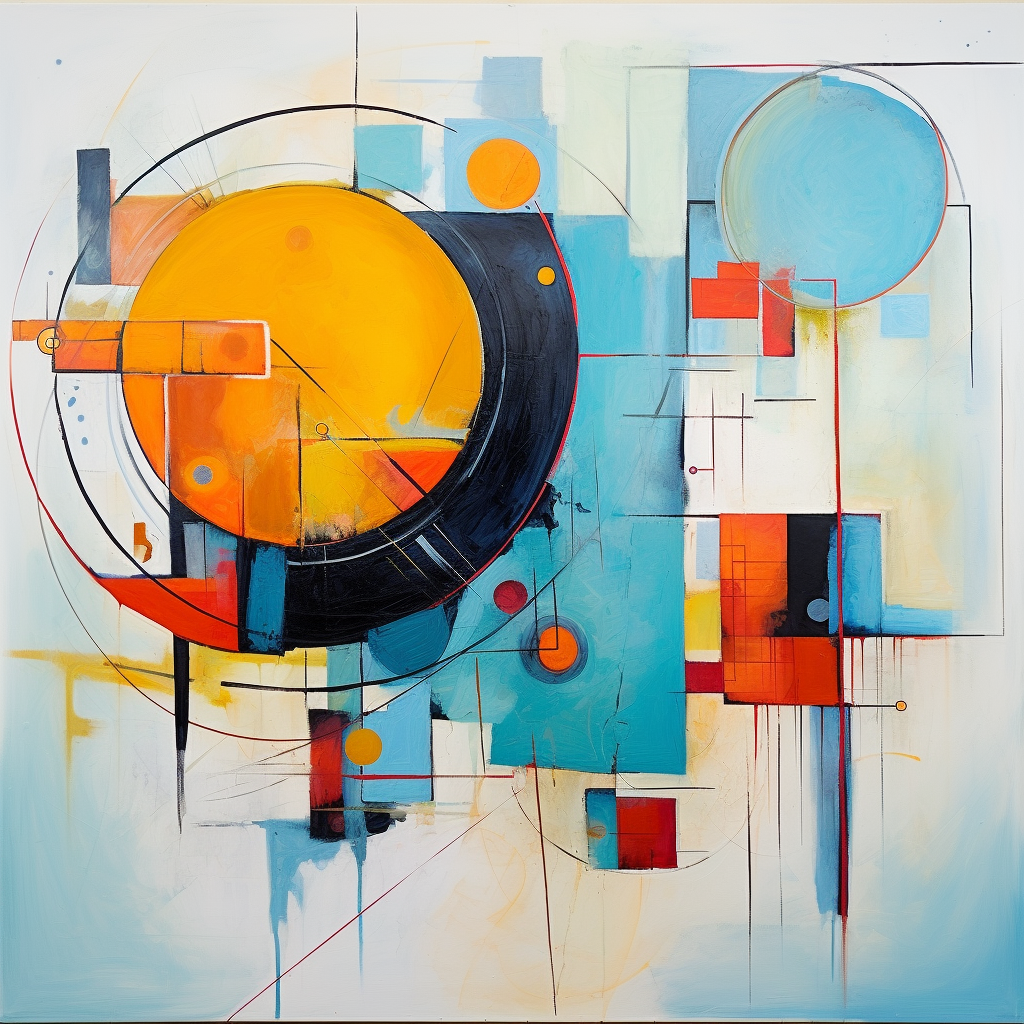
AI or Not was also successful at identifying more photorealistic Midjourney-generated images, such as this photorealistic aerial image of what is supposed to be a frozen Lake Winnipeg in Manitoba, Canada.
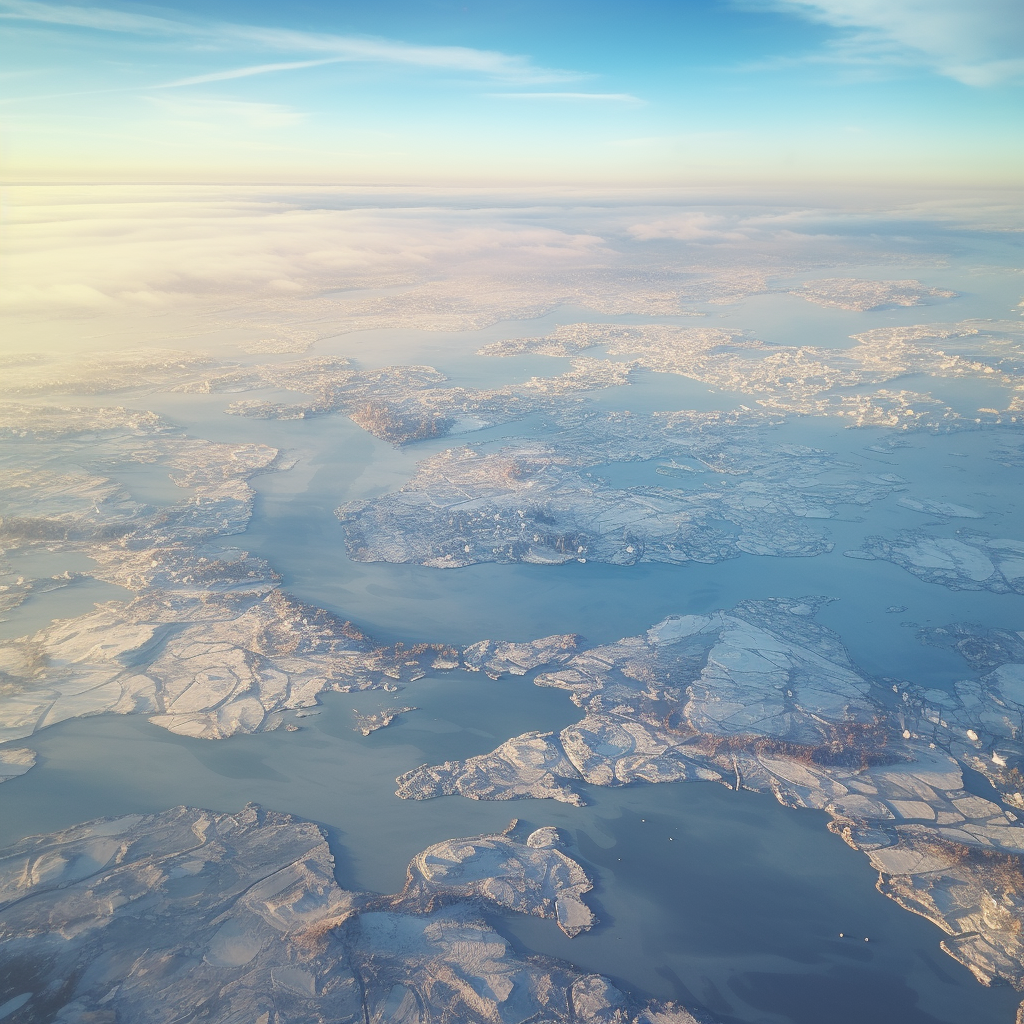
It also successfully identified AI-generated realistic paintings and drawings, such as the below Midjourney recreation of the famous 16th-century painting The Ambassadors by Hans Holbein the Younger.
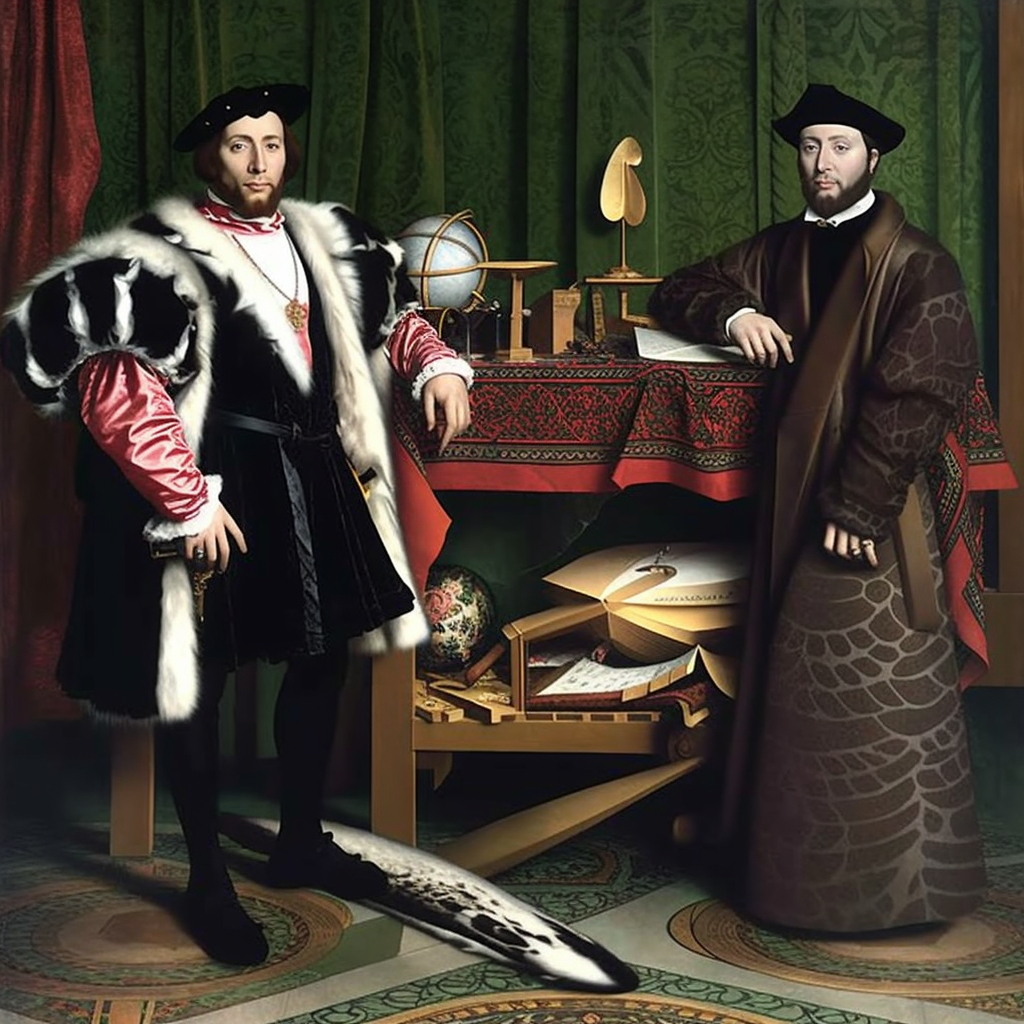
The real painting is exhibited in the National Gallery in London, UK.
Overall, AI or Not correctly detected all 100 Midjourney-generated images it was originally given.
The tool also maintained a high detection rate for real images although it also produced false positives: incorrectly identifying real images as having been generated by AI.
AI or Not successfully identified the following real images:
- 20 images taken by Bellingcat contributor Dennis Kovtun
- 20 paintings by various artists from the Renaissance period
- 19 out of 20 abstract paintings
- 10 of 10 movie stills
- 10 out of 10 animation stills
- 10 out 10 video game screenshots from the late 2000s and early 2010s
AI or Not produced some false positives when given 20 photos produced by photography competition entrants. Out of 20 photos, it mistakenly identified six as having been generated by AI, and it could not make a determination for the seventh.
All the photographs that AI or Not mistakenly identified as AI-generated were winners or honourable mentions of the 2022 and 2021 Canadian Photos of the Year contest that is run by Canadian Geographic magazine. It was not immediately clear why some of these images were incorrectly identified as AI.
Generally, the photos had a high resolution, were really sharp, had striking bright colours and contained a lot of detail. Several had unusual lighting or a large depth of field, and one was taken using long exposure.
Based on the above test, we concluded that AI or Not is quite good at identifying real images — it successfully identified real paintings and drawings and most real photographs, though it may struggle with some high-quality photos.
While AI or Not is, at first glance, successful at identifying AI images, there’s a caveat to consider as to its reliability.
AI or Not: Compressed and Watermarked Images
Every digital image contains millions of pixels, each containing potential clues about the image’s origin.
But what happens if a chunk of this valuable data is lost or distorted? During the first round of tests on 100 AI images, AI or Not was fed all of these images in their original format (PNG) and size, which ranged between 1.2 and about 2.2 megabytes. When open-source researchers work with images, they often deal with significantly smaller images that are compressed.
When an image is resized or distorted, or when its resolution is lowered, the pixels in it are altered and the “digital signal” that helps a detector identify the origins of the image is lost, Kevin Guo, the founder of image detection tool Hive, told the New York Times.
Hive provides deep-learning models for companies that want to use them for content generation and analysis, which include an AI image detector. It also has a free browser extension, but the extension’s utility for open-source work is limited. It was “unable to fetch results” on Telegram, while a small pop-up window showing the probability that an image is AI-generated did not open on X, the social media site formerly known as Twitter. The window did open on Facebook.
To test how well AI or Not can identify compressed AI images, Bellingcat took ten Midjourney images used in the original test, reduced them in size to between 300 and 500 kilobytes and then fed them again into the detector.
This produced mixed results. AI or Not falsely identified seven of ten images as real, even though it identified them correctly as AI-generated when uncompressed.
For example, when compressed, this Midjourney-generated photorealistic image of a grain silo appears to be real to the detector.
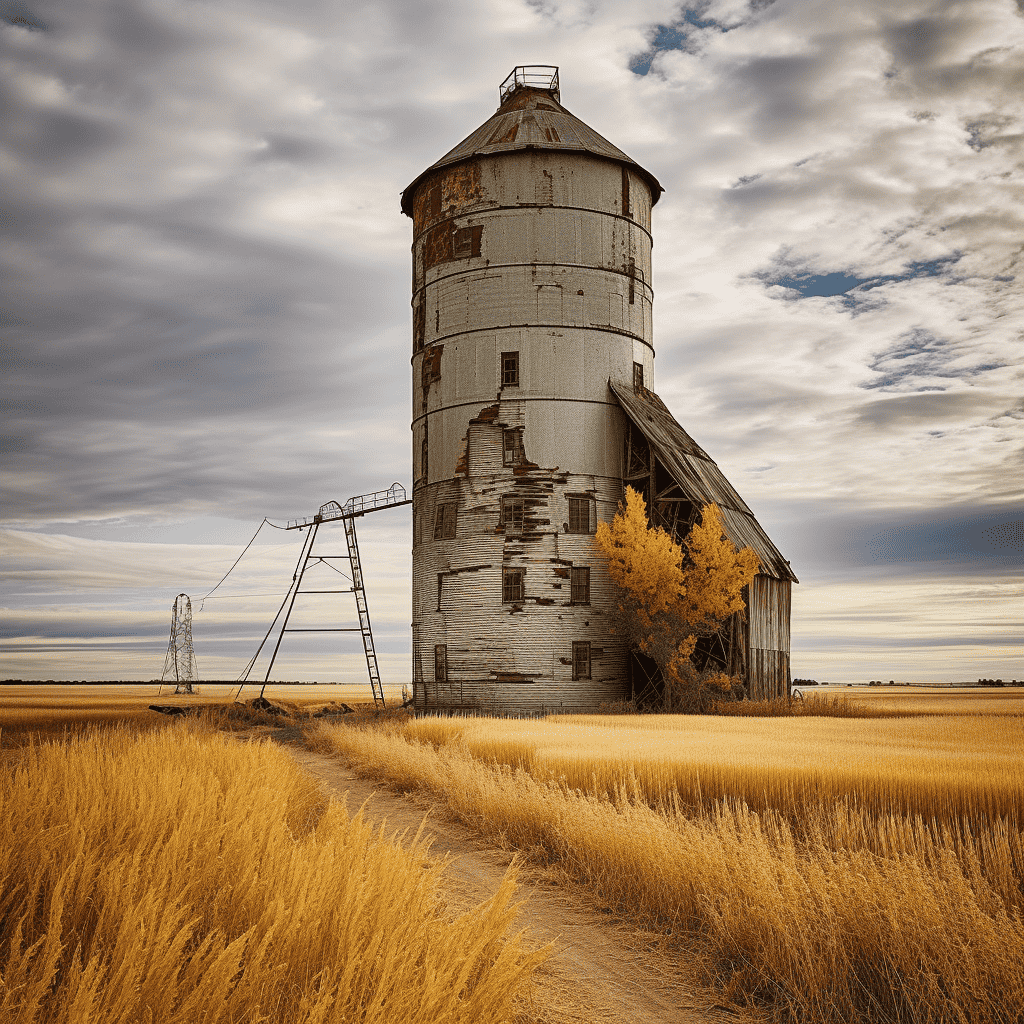
Out of ten compressed images, seven were photorealistic. AI or Not was particularly unimpressive with them — it falsely identified all seven as the work of a human. It fared better with drawings and paintings: when given three, correctly identified them, even when compressed.
With real compressed images, AI or Not fared better. It incorrectly identified two out of three paintings as AI-generated. However, it successfully identified six out of seven photographs as having been generated by a human. It could not determine whether an AI or a human-generated the seventh image.
Bellingcat also tested how well AI or Not fares when an image is distorted but not compressed. In open source research, one of the most common types of image distortions is a watermark on an image. An image downloaded from a Telegram channel, for example, may feature a prominent watermark.
Bellingcat took ten images from the same 100 AI image dataset, applied prominent watermarks to them, and then fed the modified images to AI or Not. The images were not compressed.
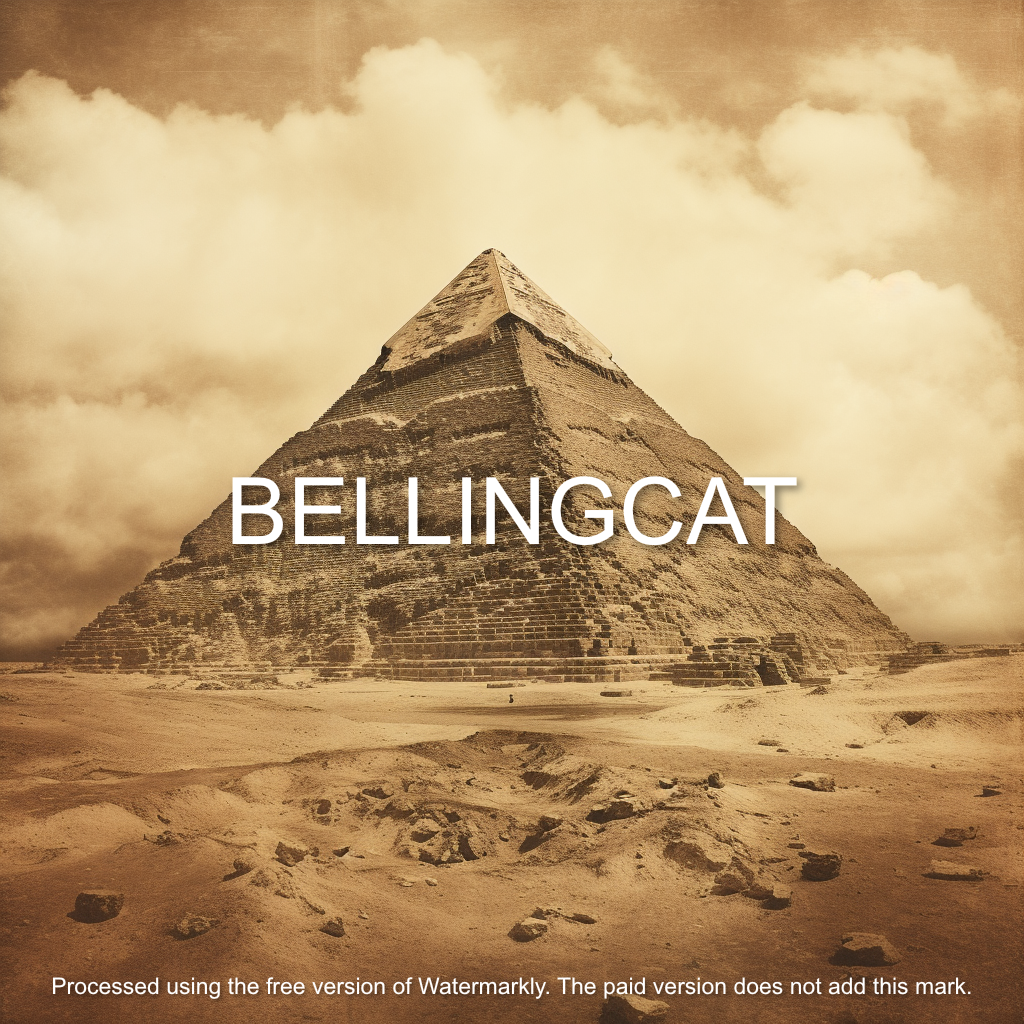
AI or Not successfully identified all ten watermarked images as AI-generated. The watermarks did not throw the detector off.
Based on this sample set it appears that image distortions such as watermarks do not significantly impact the ability of AI or Not to detect AI images. Compression, on the other hand, plays a significant role. The larger the image’s file size and the more data the detector can analyse, the higher its accuracy.
Great or Dud?
AI or Not appeared to work impressively well when given high-quality, large AI images to analyse. Its performance seems to be unaffected by watermarks.
It maintained a good success rate with real images, with the possible exception of some high-quality photos. It also worked well with compressed real images.
However, the positive results with real images are tempered by a comparatively unimpressive performance with compressed AI images. This point in particular is relevant to open source researchers, who seldom have access to high-quality, large-size images containing lots of data that would make it easy for the AI detector to make its determination.
Instead, they often work with significantly compressed images. The fact that AI or Not had a high error rate when it was identifying compressed AI images, particularly photorealistic images, considerably reduces its utility for open-source researchers. While AI or Not is a significant advancement in the area of AI image detection, it’s far from being its pinnacle.
Bellingcat is a non-profit and the ability to carry out our work is dependent on the kind support of individual donors. If you would like to support our work, you can do so here. You can also subscribe to our Patreon channel here. Subscribe to our Newsletter and follow us on Twitter here and Mastodon here.